The Ultimate Guide to Data Quality Management: A Make-or-Break Factor for Every Organization
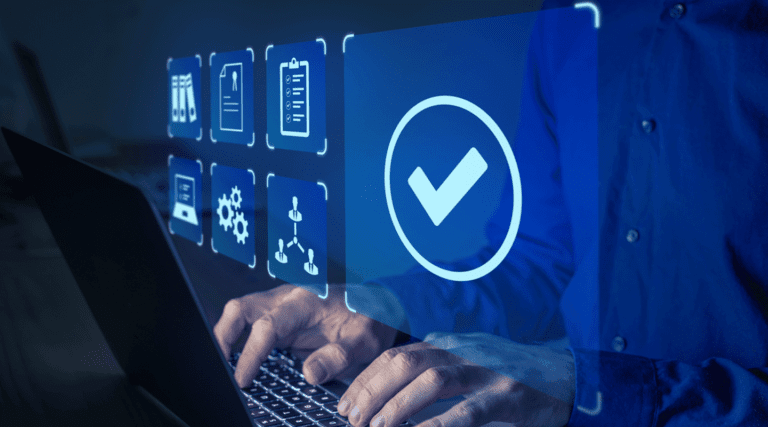
Funding the right programs to end poverty around the world. Conducting exit polling for national elections in Mexico. Measuring successful approaches to community nursing in South Carolina. Or even using a CRM system for your business.
For problems local and international, for the greater good or for the good of your organization, using high-quality data is essential. It forms the bedrock of processes, workflows, and studies that influence high-level business decision-making, far-reaching policies for social good, and real human lives on the ground. But what exactly defines this vital resource that so influences the world?
At its most essential level, high-quality data is accurate data.
“If you collect data to measure ‘x,’ did you accurately measure ‘x?’” says Dr. Christopher Robert, founder of SurveyCTO. “If a respondent answers ‘y,’ is ‘y’ the response that’s actually recorded? Again: is the data accurate?”
This is the key tenet of high-quality data, says Dr. Robert. He notes that notions of “quality” become more multifaceted when you bring in other concepts, like “research quality” and “insight quality.” Are the right questions being asked? Are the right populations being sampled? Is the research design correct? Is the statistical inference done properly? All of these things have an impact.
But at its core, high-quality data is accurate data. And it’s vital for organizations to ensure the data they’re collecting meets this essential standard, lest their own operations — and the communities they serve — suffer as a result.
While collecting high-quality data can be an expensive and time-consuming prospect for even the world’s biggest organizations, this guide will instruct readers on how to do so economically, and with the academic rigor necessary to ensure they fulfill their respective missions.
In this guide, you’ll learn:
- The false (and dangerous) assumption many organizations make about their data
- How bad data can crater workflows, processes, studies, and policy
- The three main pitfalls when collecting high-quality data
- How the world’s top organizations and academics avoid these pitfalls in a cost-efficient way
- How one organization uses high-quality data to fulfill its mission
- How to deal with issues in eliciting accurate information
- Where the future of data collection is headed
- Key takeaways about collecting high-quality data
The quality of your data matters. Here’s why.
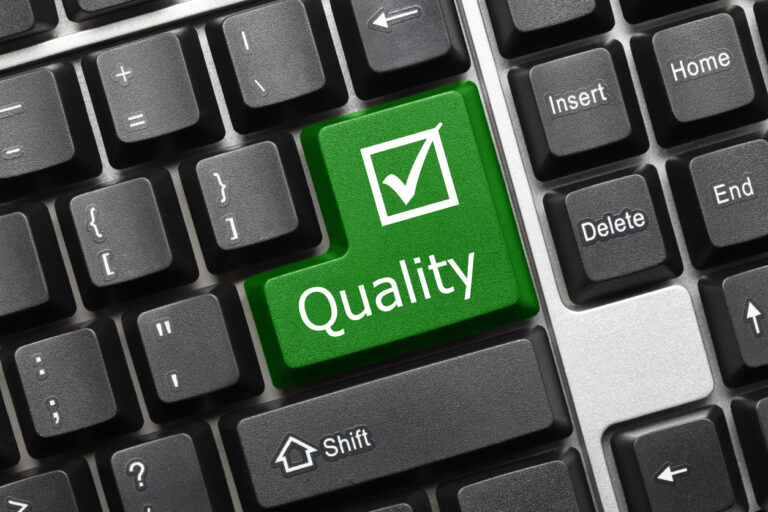
There’s a false (and dangerous) assumption many organizations make about their data.
High-quality data is crucial for solving both local and international problems, as it forms the basis for accurate decision-making in fields ranging from community nursing and electoral polling to poverty alleviation.
Use high-quality data, and you answer your most important questions faster. NGOs and government agencies can find out which approaches positively impact public health, and focus their efforts there. Is this tuberculosis program working? How about this microfinance program? Which of the households in this village are below the poverty line — and therefore qualify for assistance?
But there’s often an implicit trust that the information organizations are working with is of a high quality. Data-based processes and procedures — in nearly every profession — are built around the assumption that data is 100% accurate.
This is not always the case, says Lawrence Li, President and CEO at Dobility, Inc.
“Acquiring high-quality data is not the default setting in data collection processes,” says Li. “For instance, if you dispatch a team to collect survey data, those surveys can be poorly designed or confusing, making it difficult for respondents to provide accurate information.”
Poor data can lead to misleading insights, wasted resources, and flawed policies. It can upend workflows — and even lives.
Bad data can crater workflows and serve as the basis for poor organizational decision-making.
Organizations that use centralized Enterprise Resource Planning (ERP) software like SAP, or Customer Relationship Management (CRM) software like Salesforce, are building processes powered by data. And the quality of that data matters.
“Modern software is essentially a series of wrappers on top of databases,” says Li.
There are many database workflows where the end user is performing a data entry or data collection task, even though it’s not typically described that way.
This brings us to a crucial point: if surveys in social sciences have faced issues with data quality for decades, what does that imply for the data in our CRM systems? These are the same systems we use to allocate hundreds of millions of dollars in budgets or to make decisions about product designs.
“Data quality is not just an academic problem,” says Li. “It’s a problem for everyone.”
Academics may have been more rigorous in identifying it first, but the challenge is only beginning to gain broader recognition. It’s similar to how Institutional Review Boards have treated Personally Identifiable Information (PII) as highly sensitive for years, yet regulations like the EU’s GDPR are just now coming into play. Data quality is a much broader, often unrecognized problem.
“What SurveyCTO offers is a toolkit that addresses this issue for a larger audience,” says Li.
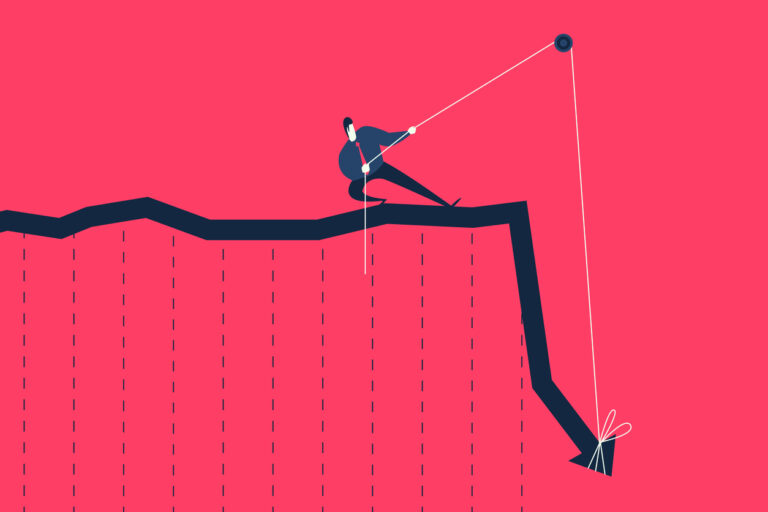
Bad data can negatively impact human lives.
In addition to hindering workflows, bad data can impact policies meant to help struggling populations, says Carlos Bohm Lopez, Data Associate at Innovations for Poverty Action. IPA’s mission is to improve the lives of people living in poverty. They do so by generating more evidence on topics related to poverty, like health, agriculture, and financial inclusion, and then getting that evidence to policymakers.
“If you don’t have good data, then your evidence is not good,” says Lopez. “You might be making the wrong decisions, and not improving the lives of hundreds, thousands, or even millions of people.”
But obtaining accurate data can be difficult and costly. Consider the budget allocated to the U.S. Census: even with billions of dollars spent and a concerted effort to reach people through door-knocking campaigns, the coverage of these campaigns is still around 60%. Non-responses are common, and there are other issues like incorrect addresses and unregistered individuals.
Despite the difficulty and cost of obtaining the right data, our systems often proceed as if we’re dealing with perfect data sets. But high-quality data is not only preferable, it’s an absolute necessity — to workflows, processes, studies, organizations, and real people on the ground.
Key takeaways from this section:
- Organizations often implicitly trust that their data is of high quality, a potentially dangerous assumption.
- Acquiring high-quality data is not the default setting in data collection processes. Poor survey design, for example, can lead to inaccurate data.
- Poor data quality can cause misleading insights, wasted resources, and flawed policies. It can also disrupt workflows and organizational decision-making.
- Software like SAP and Salesforce are powered by data, emphasizing the importance of collecting high-quality data in everyday workflows.
- Bad data can also have real human consequences, affecting policies aimed at helping vulnerable populations.
The most common traps in data collection
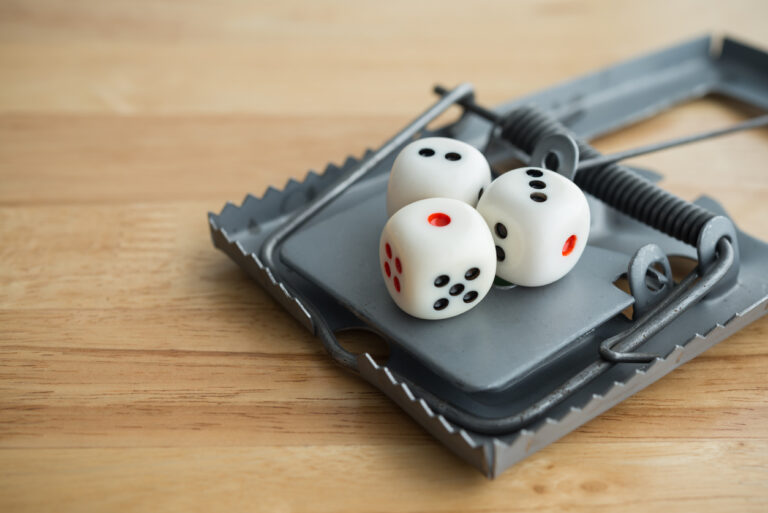
Everything you do can have implications for data quality.
If a data collector is assigned to conduct a random sampling within a specific area and they accidentally step two blocks outside of that designated area, they could inadvertently over-sample a location where another team member is also conducting sampling.
Such a seemingly innocent mistake could lead to significant issues in the conclusions drawn later on. This is especially true in places where data collectors don’t have the luxury of tools like Google Maps or comprehensive data coverage.
Another example would be personal biases. For example, a data collector may have a bias against believing that children can tolerate pain, and also be conducting a medical survey. If they underrate children’s pain levels on a Likert scale, that again affects the quality of the data collected.
The challenge of collecting good data is substantial, even for large organizations.
Significant resources are dedicated to ensuring data quality. The core of data quality is the need for reliable, accurate data. However, achieving this is no simple task; it often involves labor-intensive processes that aren’t always executed perfectly.
Take, for example, the budget of the U.S. Census — and their 60% coverage.
“The default state in the world is that it’s confusing and collecting data is hard,” says Li. “Meanwhile, the way we parse the world assumes that data quality is perfect.”
Pitfalls in data collection are numerous.
“SurveryCTO’s users are typically individuals who are trying to measure various phenomena in the world,” says Dr. Robert. “They might be looking to answer questions like, ‘is this tuberculosis program effective?’ or ‘which households in this village are below the poverty line and qualify for assistance?’ or even ‘is this microfinance program achieving its intended goals?’”
Again, there are numerous challenges in answering these types of questions, starting with the research methods employed. Considerations also include the survey design, the sample size, and whether the study is a randomized control trial to establish causality or simply a descriptive survey.
Plus, humans collecting the data can make mistakes.
“There are a dramatic number of ways in which even well-meaning humans collecting data in the world don’t follow research protocols,” says Dr. Robert. “Managing for that is what SurveyCTO focuses on — being able to facilitate direct digital data collection, but then also facilitating very robust methods of controlling for quality of data collection in the field when people aren’t being observed.”
Indeed, there are numerous potential traps in data collection. But a few are more common than others.
Common Trap 1: Enumerator effects
Enumerator (or interviewer) effects are defined as inconsistent practices among the people who collect data. They’re the most prevalent issue in data collection, says Li.
“This isn’t necessarily because interviewers are unethical or not doing good work. The biggest impact usually comes from the interview itself.”
In the field, enumerator effects can manifest in various ways — from questions that guide respondents toward particular answers, to inputting answers incorrectly, to fabrication of data in rare cases. Is the interviewer asking the questions in the way they’re supposed to, every single time, with every respondent? This can be hard to track when no one is observing them and no supervisors are nearby.
Enumerators are also sensitive to their respondents’ needs, as people generally don’t want to spend hours being interviewed, says Dr. Robert.
“In a well-meaning attempt to expedite the process, an interviewer might think, ‘I’m not going to go through the entire explanation behind this question,’ or ‘I won’t even ask this question at all because the answer seems obvious, so I’ll answer it for them.’”
Alternatively, an interviewer might think that a particular type of answer will add an extra hour to the survey, and may subtly steer the respondent in another direction. For instance, saying, “No, you haven’t taken a loan; maybe it was 13 months ago, not 12, that you took the loan,” just to avoid triggering a longer line of questioning.
There are a multitude of ways — even when humans are well-meaning — in which data collection doesn’t strictly follow research protocols. Managing these factors involves both the ability to facilitate direct digital data collection, and implementing robust methods to control for data quality in the field, particularly when people are not being observed.
Common Trap 2: Sample effects
Sometimes, organizations fall into the trap of collecting data from convenient but unrepresentative samples, which can skew the data and offer misleading conclusions.
Whether it’s an overreliance on urban populations for rural studies or the exclusion of marginalized communities, poor sample selection can have far-reaching implications for the quality of data and subsequent policymaking.
“I’d venture to say sample effects are the second-biggest [trap],” says Li.
Common Trap 3: Instrument effects
Poorly designed survey instruments can lead to a different but equally problematic form of bias: instrument effects.
This can range from leading questions and ambiguous phrasing to poorly designed response scales. These design flaws can inadvertently guide respondents toward particular answers, thereby reducing the reliability of the data collected.
“Did you write the survey well or not?” asks Li. “Obviously, you don’t want to write questions that could be interpreted 10 ways by 10 people.”
Key takeaways from this section:
- Every action taken during data collection can affect data quality.
- While data quality is assumed to be perfect when interpreting the world, the reality is far from it. Collecting good data is a significant challenge, even for large organizations, requiring substantial resources and labor-intensive processes.
- Variability in data collection practices among enumerators is the most common data quality trap; even well-meaning interviewers can fail to follow research protocols.
- Collecting data from unrepresentative samples can lead to misleading conclusions, such as over-relying on urban populations for rural studies.
- Poor survey design, including leading questions and ambiguous phrasing, can also bias the results.
How to overcome these common traps
SurveyCTO offers a robust suite of features designed to tackle the common pitfalls in data collection. These features address specific issues as well as enhance the overall statistical quality of data at the post-collection stage.
The Trap: Enumerator effects
The solutions: Audio capture, photo capture, GPS, monitoring, and field-level validation.
One ultimate source of truth in data collection is an audio recording, says Dr. Robert.
Audio recordings of interviews are incredibly helpful because if questions arise about what transpired in an interview, there’s a source of truth to consult. GPS positions and timing data—such as how much time was spent on each question and the path taken through the survey—can also be invaluable in that respect.
SurveyCTO aims to address the myriad problems surrounding data quality by making it quick and easy to initiate monitoring from the first day of data collection. There are essentially three types of monitoring.
The first type are automated quality checks, which are statistical in nature. For example, if one interviewer consistently gets a “yes” answer to a specific question 80% of the time, while all other interviewers only get a “yes” 30% of the time, it suggests that something might be off. It could be a training issue or perhaps even a case of fraud. These statistical checks, sometimes called high-frequency checks, are built into SurveyCTO.
“So, you don’t need a data analyst to write a bunch of special code, which might take weeks,” says Dr. Robert. “In order to be able to perform these kinds of statistical checks, we built them into the software. They’re there and ready to go from the first day.”
The second type of monitoring involves the need to investigate specific cases, whether flagged randomly or based on prior statistical checks. SurveyCTO has created tools that allow users to delve into an interview closely. They can review GPS positions, listen to audio recordings, and examine the time spent on each question. They can even view photos; for instance, if it’s reported that a structure has a metal roof, they can check the corresponding photo to verify.
The third form of monitoring is examining data in aggregate. This involves looking at broader patterns in the data as it comes in, which can help identify potential issues. For example, a user might want to view a map showing the locations where different interviewers conducted their interviews. Or they might examine a cross-tabulation to understand the breakdown of loans by gender.
In summary, SurveyCTO provides the tools necessary for three different kinds of monitoring: automated statistical checks, the ability to investigate individual cases closely, and the option to review aggregated data. These tools facilitate navigating between all three types of monitoring to ensure the highest data quality.
Another simple but effective measure against this is field-level validation. For example, ensuring that a system rejects implausible values like an age input of 1000. Such measures not only restrict the enumerator’s ability to accidentally put the wrong data in, but also serve as a mechanism to flag those who might be entering nonsensical information.
“SurveyCTO performs statistical quality control using what’s referred to as paradata,” says Li. “No personally identifiable data from the primary dataset is needed; you just require the background information, or ‘light shading data,’ captured by the device. This allows you to discern what enumerator behavior is normal versus abnormal.”
In practical terms, this means users can determine whether an enumerator is genuinely conducting interviews in the field or merely sitting in a coffee shop. Furthermore, SurveyCTO offers a review and corrections workflow, ensuring that data isn’t accepted until thoroughly vetted. Users also have the flexibility to decide the percentage of submissions that need to be reviewed.
The Trap: Sample effects
The Solution: Case management rules
SurveyCTO has developed case management tools designed to help users streamline entity-based data collection, which tracks data collection subjects like individuals, facilities, or households longitudinally.
These tools focus on maintaining accurate data on the subjects — respondents, schools, clinics, or other entities — you’re keeping track of over time. This is particularly useful in fields like health services and census-type services. The goal is to ensure that the user is reaching the right people, and not just reaching them, but reaching them multiple times for longitudinal studies. It’s crucial that the data recognizes these individuals as the same people over multiple interactions.
This feature set is designed to help address issues related to sample selection.
The Trap: Instrument effects
The solutions: Translation tables (and in the future, AI)
SurveyCTO offers translation tables, which assist users in maintaining translations within the structure of their interviews.
They’re also in the early stages of leveraging generative AI for something known as cognitive interviewing. Cognitive interviewing involves creating a survey and then interviewing a subset of people to understand their thought process regarding the questions. This ensures that they interpret the questions as intended and that their answers are consistent.
Normally, this process is expensive, says Li, but it’s much easier with a large language model. With LLM, you can simulate limitless personas who will not only answer the survey questions but also provide a paragraph explaining their thought process behind each answer. This mimics the methodology of cognitive interviewing.
While you can currently use something like GPT for this purpose, the SurveyCTO team is exploring several potential ways to improve on and integrate the capability into its platform. This is more of a forward-looking feature, and not yet available today.
Academic rigor: Why Nobel Prize winners use SurveyCTO.
SurveyCTO is exclusively used by Innovations for Poverty Action and J-PAL, the two leading development economics organizations in the world. The co-founders of J-PAL won the Nobel Prize in Economics in 2019.
“The most rigorous methodologists in social sciences use SurveyCTO,” says Li.
In the broader development world, SurveyCTO is utilized by organizations like Oxfam and the World Bank. Within the World Bank, a group called DIME (Development Impact Evaluation) uses SurveyCTO. These impact evaluators are among the most academically rigorous measurement experts in international development.
“Between IPA, J-PAL, and DIME, everyone in the development world understands that SurveyCTO is the most methodologically rigorous tool available,” says Li.
Using audio recordings as a tool to help manage data collection teams.
Often, when we talk about audio recordings, the immediate thought is quality control—drilling down to understand what happened in an interview to provide feedback to the interviewers, for example. But there’s another, perhaps less obvious, way that audio recordings have been effectively used: as a collaborative learning tool.
Data collection teams often have weekly meetings where they listen to a random selection of interviews. This serves as a learning opportunity for everyone, whether they’re new or experienced team members. Knowing that your interview could be played in front of your peers creates a high standard that team members want to reach.
This approach also provides teams with a way to learn from their shortcomings in a more collaborative manner. This isn’t about quality managers listening to recordings and giving performance reviews. Instead, it’s a peer-to-peer learning experience.
Audio recordings are incredibly valuable even if users don’t have a dedicated quality team to listen and follow up. They offer a way to make interviews transparent and serve as an effective tool for continuous learning and oversight within the team.
Key takeaways from this section:
- SurveyCTO offers a comprehensive set of features to address common challenges and improve the statistical quality of data collected.
- It tackles data quality traps, such as enumerator effects, sample effects, and instrument effects with specific solutions like audio capture, photo capture, GPS, monitoring, and field-level validation.
- Audio recordings are an important source of truth in data collection, useful for addressing questions that arise post-interview.
- SurveyCTO offers three types of monitoring: automated statistical checks, entity-specific investigation, and aggregate data review.
- Automated statistical checks, sometimes called high-frequency checks, are built into the software and don’t require special code by a data analyst.
- Case-specific monitoring allows users to review GPS locations, listen to audio recordings, and examine time spent on each question, among other features.
- Aggregate monitoring enables users to look at broader patterns in the data to identify potential issues, such as the geographic spread of interviews or gender breakdowns of loans.
- Field-level validation restricts implausible values, serving as both an error-prevention measure and a flag for possible fraudulent behavior.
- SurveyCTO uses paradata for statistical quality control, which does not require personally identifiable data and helps differentiate normal from abnormal enumerator behavior.
- Case management rules are designed to keep track of individuals longitudinally, particularly useful in fields like health services and census services.
- SurveyCTO offers translation tables to assist with maintaining the quality of translated interviews and is also exploring the use of generative AI for cognitive interviewing.
- The platform is trusted by leading development economics organizations, including Innovations for Poverty Action and J-PAL. In the broader development world, SurveyCTO is also used by organizations like Oxfam and the World Bank, particularly within its Development Impact Evaluation (DIME) unit.
- Audio recordings can serve as collaborative learning tools for data collection teams, offering a peer-to-peer approach to quality management and continuous learning.
How one organization uses SurveyCTO to tackle some of the world's most pressing issues
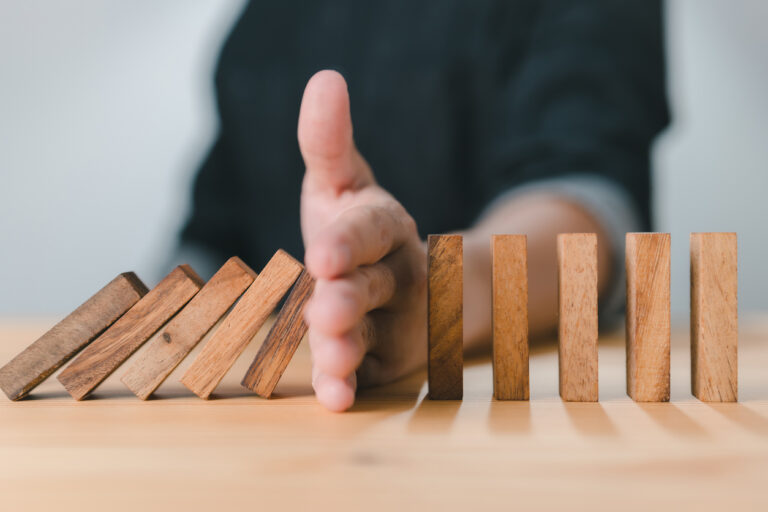
Innovations for Poverty Action’s mission is to tackle poverty. Operating across various domains like agriculture, health, financial inclusion, and more, IPA aims to generate evidence-based insights that inform policy.
To realize this mission, the organization prioritizes high-quality data collection. As Carlos Bohm Lopez, a Data Associate from IPA, points out, “If you don’t have good data, then your evidence is not good. You might be making the wrong decisions, and not improving the lives of hundreds, thousands, or even millions of people.”
The quality of IPA’s data impacts real human lives on a large scale.
IPA’s primary customers are principal investigators. These are esteemed researchers from universities, government agencies, or multilateral organizations, usually focused on topics related to poverty. They set the research agenda and formulate questions, then hire IPA to ensure smooth data collection, which is their area of expertise.
Once they find certain effects and establish the evidence, they connect with the appropriate people in the government. Often, the government decides to roll out the initiative at a national level. The work has the potential to impact many people.
Whether their client is from the government or a respected researcher, these individuals often have strong connections to policymakers. Sometimes they even transition into policy-making roles themselves later on.
Bad data can erode organizational trust and negatively affect policy.
As discussed, data quality can be compromised in multiple ways.
A poor research design could result in questions that are not understandable to respondents or are simply badly formulated. Additionally, data can be flawed if enumerators misunderstand the questionnaire, or if respondents fill it out incorrectly. Programming errors can also contribute to poor data quality.
“Let’s say there’s a question that is only asked to women,” says Lopez. “And then let’s say our respondents are women, but then this question doesn’t get turned on because there was a problem with the programming. That’s bad data. You’re missing out on [an answer] you need.”
These errors can have a range of consequences. In terms of funding, if key questions haven’t been asked, IPA may need to revisit the field to collect that information. This not only involves additional financial resources but also the effort to reconcile the new data with the old. Such lapses can erode the trust of principal investigators who rely on IPA for data collection.
Looking beyond the context of IPA, bad data can lead to misguided policy decisions. For instance, if data suggests that a particular program is highly effective when it actually isn’t, then the money being spent on that program is mis-allocated. Conversely, if a genuinely effective program is evaluated using flawed data, its true worth may not be recognized, causing a missed opportunity to scale it up.
How IPA uses SurveyCTO to achieve their mission.
For an organization like IPA that grapples with poverty issues worldwide, high-quality data is not just an add-on; it’s a cornerstone.
Poor data quality could lead to ineffective programs and mis-allocated resources, hindering the organization’s ability to affect real change. On the other hand, high-quality data provides reliable evidence that reaches policymakers, which is an essential aspect of IPA’s work.
Lopez says that SurveyCTO is not just another tool for IPA; it’s the most recommended way of data collection within the organization.
“It’s easy to understand, easy to handle, and it has so much functionality. Also, it has a fantastic help and support center.”
In the diverse and challenging settings where IPA operates, flexibility is crucial. SurveyCTO offers precisely that.
“We have so many projects in so many different countries with so many different contexts,” Lopez says. “We need to use case management and server data assets.”
A recent development he highlighted is offline publishing, which allows data sets to be transferred without an internet connection. This is particularly useful for enumerators working in isolated regions. The versatility of SurveyCTO also extends to its real-time data monitoring capabilities.
“I love the ability to connect the incoming data to Google Sheets, so you can have dashboards right away,” he adds. The tool also allows for quality checks and dashboard creation directly within the server as the data arrives. “All these tools and services allow us to collect better data. SurveyCTO can be as complex or simple as you need.”
Given the high stakes of IPA’s mission to alleviate poverty, utilizing a robust tool like SurveyCTO helps ensure that their data—and thus their impact—is of the highest quality.
Key takeaways from this section:
- Innovations for Poverty Action (IPA) is committed to tackling poverty across various domains such as agriculture, health, and financial inclusion by generating evidence-based insights to inform policy.
- High-quality data collection is a priority for IPA, as it directly influences the quality of evidence and, by extension, the impact on human lives on a large scale.
- Poor data quality can have serious consequences, including eroding trust with principal investigators and leading to misguided policies. The quality of data can be compromised through poor research design, enumerator misunderstandings, or programming errors.
- To maintain high-quality data, IPA uses SurveyCTO as their most recommended way of data collection within the organization.
- SurveyCTO offers the flexibility that IPA needs, given the diverse and challenging environments in which it operates. Features like offline publishing and real-time data monitoring are particularly useful for IPA’s work.
- The tool is also praised for its ease of use, functionality, and robust support system.
- The use of SurveyCTO aligns with IPA’s mission to generate high-quality data, thereby ensuring that their mission of alleviating poverty is realized.
Addressing challenges in eliciting accurate information
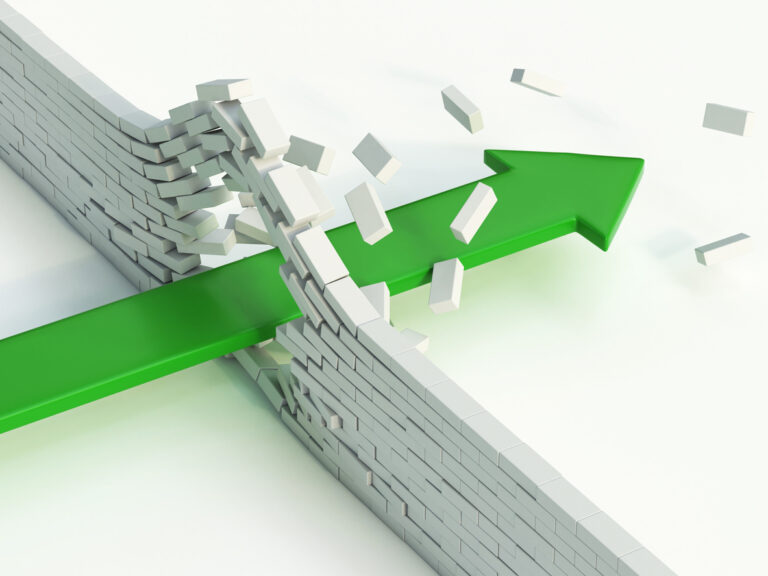
Navigating the complexities of human behavior to collect accurate information is a major challenge in data collection. Factors such as social desirability, literacy, and sensitive topics can significantly skew data quality.
This section explores how SurveyCTO has tools to counteract these issues.
Defining the social desirability effect.
The social desirability effect refers to the tendency of survey respondents to answer questions in a manner that will be viewed favorably by others. Essentially, people are inclined to provide socially acceptable or “desirable” responses, rather than being completely honest, especially when asked about sensitive or controversial topics.
This can skew data and create a bias in research findings, as the collected information may not accurately reflect the respondents’ true thoughts, feelings, or behaviors. The effect can be a significant concern in fields like psychology, sociology, and market research, where understanding true preferences and behaviors is crucial.
People may be hesitant to provide honest answers to questions about topics like abortion or criminal activity, as they often opt for answers that seem more socially acceptable or less shameful. This is particularly true in religious cultures.
SurveyCTO’s countermeasures.
To address this data quality issue, SurveyCTO provides robust options to conduct interviews that can work through these problems.
- Discreet Response Mechanisms: In situations where respondents may not feel comfortable being honest verbally, SurveyCTO allows the interviewer to hand the respondent a tablet with questions presented visually. The interviewer then turns away, enabling the respondent to select their answers privately.
- Audio Assistance: If literacy is a concern, SurveyCTO offers the option of providing earphones so that the respondent can listen to the questions and respond by clicking on images on the screen, maintaining their anonymity.
- Specialized Tools: In educational settings, SurveyCTO offers specialized tools for assessing reading and math skills in children, ensuring the integrity and quality of the data collected.
- Innovative Data Collection in Agriculture: For agricultural inquiries, instead of merely asking farmers about the sizes of their fields, SurveyCTO provides the option of capturing this data more accurately. Farmers can walk the perimeter of their field while pressing a button on a device, allowing SurveyCTO to calculate the field’s exact area automatically.
Key takeaways from this section:
- Navigating human behavior is a significant challenge in collecting accurate data, with factors like social desirability, literacy, and sensitive topics potentially skewing the quality of the data.
- The social desirability effect causes respondents to provide answers they believe are socially acceptable, affecting the integrity of research across fields like psychology, sociology, and market research.
- SurveyCTO offers various tools specifically designed to mitigate the challenges posed by social desirability and other factors affecting data quality.
- These tools include discrete response mechanisms, audio-assisted features, specialized educational tools and tools for data accuracy in agricultural settings.
New horizons in data collection highlight the continued importance of data quality management
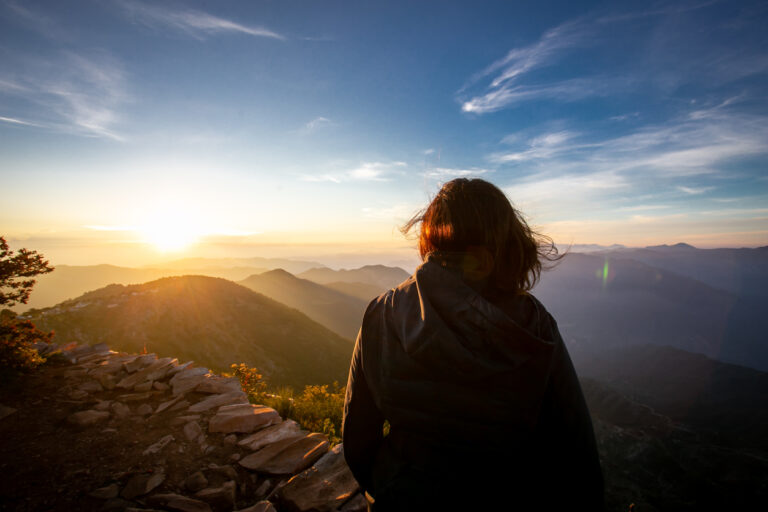
Data collection methods are not static; they are continually influenced by emerging technologies and shifting needs. In this section, we examine the areas in which the environment is changing rapidly.
M&E becomes more rigorous
Organizations are increasingly investing in Monitoring and Evaluation (M&E). M&E is the broader space that includes, but is not limited to, rigorous, academic-style methods of data collection and analysis.
For context, think about the World Bank, which funds thousands of development projects annually. They may only conduct rigorous impact evaluations on a small percentage of these projects. However, the rest are not going unmeasured; they’re using traditional M&E methods to track operational metrics, like the distribution of mosquito nets.
As M&E methodologies become more rigorous, the demand for higher-quality data collection tools like SurveyCTO increases. Organizations are transitioning from using paper and pen to research-caliber tools, especially when they need to track quality-of-life impacts over a period.
There’s also a shift towards decision-oriented M&E, which requires real-time feedback and data dashboards. This necessitates high-quality data collection platforms that can easily integrate with other software, such as Salesforce or DevResults.
SurveyCTO is a perfect fit for this transition, says Li.
We already have all the tools to ensure the data quality. We already have all the export formats and integrations. This is our chance to bring user-friendly — but super rigorous — capabilities from the research world to others doing fieldwork.
Lawrence Li.
The increasing importance of offline data collection
On a visionary level, the power of data has been proven and is currently benefiting many sectors, especially in high internet penetration countries. Hospitals have electronic records, companies optimize marketing spending, and employee happiness is monitored.
One barrier to expanding these benefits globally has been the requirement for high-quality internet connectivity. This is where SurveyCTO comes in, says Li.
“Our cohort of collection tools bridge that gap.”
Using SurveyCTO, people are now able to build electronic medical records in refugee clinics that will otherwise not have internet connectivity for two decades. Once a week, their collection devices can be synced to a cloud server, offering a fully functional, cloud-based electronic medical record system even in the most remote locations.
Areas for future innovation and the human element
“There are new opportunities for data collection all the time,” says Dr. Robert. “Over the years, we’ve seen the rise of IoT devices, satellite data, and even crowdsource platforms.”
However, the human element remains crucial for many types of data collection, says Dr. Robert, especially when it comes to lower-literacy populations. Interviews continue to be an important method for gathering valuable information. As important as new technologies are, the need to conduct interviews skillfully — and with the right tools — will persist for the foreseeable future.
Innovation in how these interviews are conducted must continue; making quality control methods more accessible and cost-effective is crucial.
In that vein, SurveyCTO has invested in using machine learning technologies to recognize more subtle patterns, whether it’s in sensor data or response patterns during interviews. These technologies allow users to look holistically at interview data, helping them identify areas for training and improvement. By using machine learning, they can continue to refine and make more efficient their quality control practices.
Key takeaways from this section:
- Organizations are increasingly investing in Monitoring and Evaluation (M&E), widening its scope beyond just academic-style methods.
- As M&E becomes more rigorous, there’s a growing demand for high-quality data collection tools like SurveyCTO. Organizations are shifting from paper-and-pen methods to research-caliber tools, particularly when tracking quality-of-life impacts over time.
- A new trend is emerging towards decision-oriented M&E, which necessitates real-time feedback and data dashboards. This requires data collection platforms that can integrate with other software systems like Salesforce or DevResults.
- SurveyCTO is well-positioned for this shift in M&E, offering all the necessary tools to ensure data quality, as well as various export formats and integrations.
- Offline data collection is becoming increasingly important, especially in regions with low internet connectivity. SurveyCTO’s collection tools are designed to meet this need, allowing for the creation of cloud-based electronic medical record systems in remote areas like refugee clinics.
- Despite the emergence of new technologies, the human element remains crucial in data collection, especially for lower-literacy populations. Interviews will continue to be a key method of data collection. Continuous innovation is needed in how interviews are conducted to make quality control methods more accessible and cost-effective.
- SurveyCTO is investing in machine learning technologies to improve data quality. These technologies allow for a more holistic view of interview data and help identify areas for training and improvement.
Conclusion: Data collection is hard work, but must be done with academic rigor — and in a cost-effective manner — for organizations to effectively make decisions and serve their stakeholders.
In this article, we’ve delved into the multifaceted world of data collection and its manifold challenges, from common traps such as enumerator, sample, and instrument effects, to dangerous assumptions most organizations make about the quality of their data.
SurveyCTO emerged as a critical tool in our discussion, offering a suite of robust features designed to tackle the inherent pitfalls of data collection. Whether dealing with enumerator effects through GPS, photo, and audio captures, or counteracting sample effects via specialized case management rules, SurveyCTO offers numerous, easy-to-use ways to ensure data quality.
Moreover, organizations like Innovations for Poverty Action (IPA) are leveraging these tools to tackle some of the world’s most pressing issues, underlining the real-world impact of data quality.
Collecting high-quality data is challenging work. It’s also crucial.
Collecting accurate and reliable data is by no means an easy task. The road to obtaining “the truth” is fraught with challenges, from the psychological tendencies of respondents to technological complexities and ethical concerns. It requires a blend of innovation, integrity, and vigilance to ensure that the data serves as an authentic reflection of reality.
“We understand how difficult this work is,” says Dr. Robert. “We understand how many challenges there can be to getting at the truth.
At the same time, the need for high-quality data — for accurate data — has never been more clear. From healthcare and agriculture to policymaking and financial inclusion, data serves as the foundation upon which critical decisions are made.
High-quality data can lead to actionable insights that can transform lives, communities, and entire nations. This is why organizations like IPA prioritize high-quality data collection, fully understanding that poor data can thwart their mission.
Key takeaways from this article:
- Organizations frequently operate under the potentially dangerous assumption that their data is inherently of high quality.
- High-quality data is not a given in data collection processes; issues like poor survey design can lead to inaccuracies. Low-quality data can have detrimental effects, leading to misleading insights, wasted resources, and flawed policies.
- Software platforms such as Salesforce rely heavily on data quality, underlining the importance of high-quality data in various organizational workflows. The impact of poor data also extends to human lives, particularly when it influences policies designed to assist struggling or vulnerable populations.
- Data quality is affected by every action taken during the data collection process, and even seemingly minor errors can have significant consequences. Even large organizations with significant resources, like the U.S. Census, struggle with data quality issues such as non-responses and incorrect data.
- Enumerator effects, which are inconsistencies in practices among those who collect data, are the most common trap in data collection. Sample effects, or using unrepresentative samples for data collection, can lead to misleading conclusions and poor policymaking. Instrument effects, such as poor survey design or ambiguous questions, can also compromise the reliability of collected data.
- SurveyCTO offers a comprehensive suite of features that address these issues and facilitate high-quality data collection, from audio, photo, and GPS tools, to automated statistical checks that can highlight anomalies in data collection.
- SurveyCTO also offers case management tools to track individuals over time, particularly useful for longitudinal studies in fields like health and census services. Translation tables to maintain translation integrity during interviews.
- SurveyCTO is developing AI capabilities for cognitive interviewing, which will allow for more accurate and consistent survey responses.
- SurveyCTO is used by organizations like Innovations for Poverty Action (IPA), J-PAL, Oxfam, and the World Bank for its methodological rigor.
- Organizations are increasingly investing in Monitoring and Evaluation (M&E), moving towards more rigorous methodologies and high-quality data collection tools like SurveyCTO.
- The work of collecting high-quality data is complex and challenging, but serves as the foundation for critical decisions across various sectors. SurveyCTO offers robust features to tackle challenges in data collection, like enumerator effects and sample effects, ensuring data quality.
Ensure a unified approach to data security
Ultimately, data security is a collective responsibility that transcends individual devices and databases. It demands a holistic organizational approach that encompasses technology, policy, and human behavior. End-to-end encryption, regular training and awareness programs, and a proactive stance toward emerging threats will enable organizations to stay one step ahead of potential risks.
Better data, better decision making, better world.