- Last Updated: January 23, 2025
Ensuring data quality is essential. Learn about the tips and tools you need to ensure data quality in your research and how to overcome some common challenges.
Strong data quality helps back up important decisions, evaluate the success of programs, and obtain funding.
How is quality assurance in data collection achieved? When the data you have collected meets your initial requirements. If the final data is not fit for your intended purpose, this can cause inaccuracies, which leads to wasted time and resources.
In this article, we’ll explain further about what good quality data is. We’ll then provide some tips you can follow to improve the quality of your data and look at common challenges you might face trying to achieve data quality. Finally, we’ll show you how tools like SurveyCTO can help ensure quality data.
What is data quality?
Simply put, data quality in field research is a measure of whether your collected data meets your research requirements. Quality field data should not have any errors, it should represent respondents accurately, and should be free of any bias.
“Good data” can mean different things for different organizations and can vary according to context. However, the following characteristics are generally accepted to represent good data quality:
- Accuracy: There should be no errors in the data.
- Relevance: Your data should only include fields that you actually need and information that you intended to collect.
- Completeness: No questions should be left partially unanswered or skipped.
- Timeliness: The timeliness of the data should fit the purpose of your research, which is most often real time.
- Consistency: There should be consistency across the questions being asked and these questions should be carefully planned according to the goals of your research.
Why is data quality important?
Important policy decisions or funding decisions are often made based on research data. Data is also often used to evaluate important work that organizations are doing.
For example, Magic Bus India Foundation, an organization that tackles childhood poverty, uses survey data to measure the efficacy of their programs and to see where changes or improvements can be made.
If data contains erroneous information, you run the risk of having to repeat all of your research. This could cost your organization or project both time and money. In more serious cases, funding or resources could be incorrectly allocated, and lives could be impacted.
To avoid all of this, you should take steps to ensure data quality and monitor it, from the planning stages and beyond.
Tips to ensure data quality in field research
1. Put a data quality assurance plan into place
A solid data assurance plan is the bedrock for data quality. This is a plan that allows you to imagine anything and everything that could go wrong during your data collection phase and put in place solutions to prevent these issues.
2. Run tests ahead of time
Testing is a core component of a quality assurance plan. Testing also lets your data collection team members offer any feedback that can ensure data quality is achieved. Performing the following tests helps catch any errors before they become costly to solve.
- Test your questionnaire on different devices. Once you’ve created your questionnaire in your survey tool, you should test it on a range of devices. This will help mitigate any technical difficulties your enumerators might have while interviewing in the field.
- Pre-test questions. Interviewing a small group of people in advance, also known as a pilot survey, helps you evaluate the strength of the survey questions and gives time to improve them before launching into your research.
- Test how your data will be exported. This will allow you to see if everything is formatted in a way that is conducive to your data quality checks and other downstream data processes.
3. Train your team
The best way to ensure consistency and get everyone working to the same standards is to train everyone in the same protocol. Even if team members have extensive experience, you should assume that they will need additional training for new projects. This will lead to greater consistency and fewer errors. Training may involve showing how to use a case management workflow, as well as mock interviews.
As part of the training process, you should also explain to the team the “why” behind what they are doing. If they feel included in the overall aims of the project, they’re more likely to be motivated to work towards a common goal.
Enumerators may even detect problems with the survey during training and this will again allow you to make corrections while it is still simple to do so.
If enumerators face challenges during a project, you should consider hosting a follow-up training session to address issues.
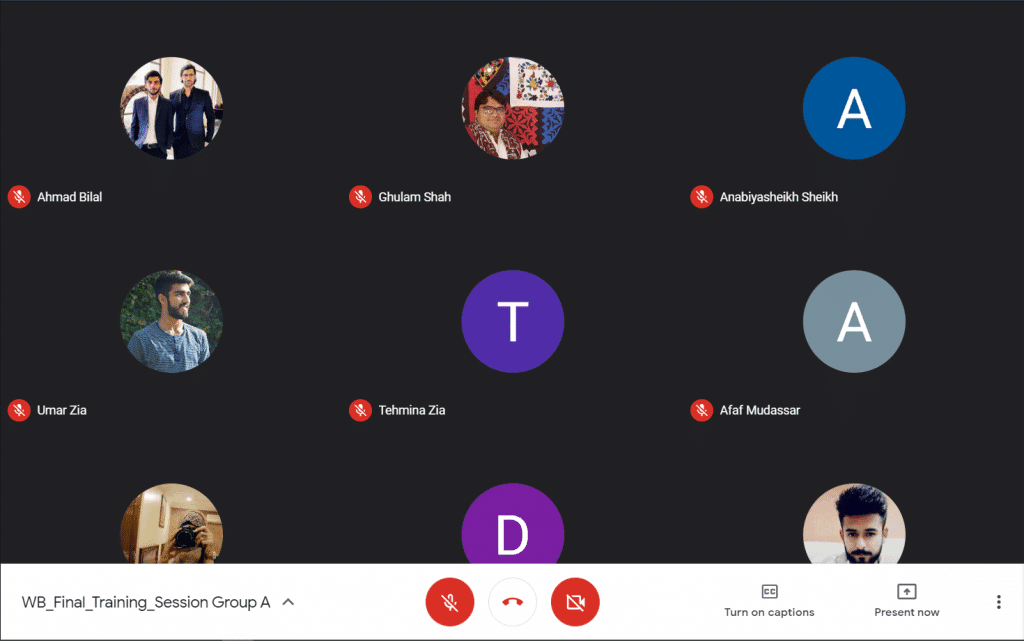
4. Improve communication between teams and with respondents
There are a number of things you can do to enhance communication within your team. A key measure is setting up channels and providing tools where team members can access information in real time.
Make sure you establish objectives for enumerators and research assistants early on, as well as feedback mechanisms that allow for feedback to flow in both directions. Enumerators, supervisors, and research assistants should also be aware of who they can contact if they need help or encounter any issues.
To improve communications with respondents, you should plan and/or budget ahead for translation issues, connectivity issues, or time zone difficulties. Remote or decentralized data collection can also heighten communication issues, but these challenges can be overcome with the right systems and planning in place.
5. Implement quality checks
When performing quality checks, errors are more likely to be flagged early in the data collection process, and will therefore be least costly. Quality checks can include automated checks, high-frequency checks, audio audits, or other types of quality checks. Whichever you choose, these checks should be planned and built into your quality assurance plan from the beginning.
Random, independent back-checks to validate survey responses are also essential. These checks verify if your data is correct, complete, whether there are any duplicates, and whether it’s consistent.
6. Get the right tools to help you monitor your data
Relying solely on manual labor to help check your data as you work is a recipe for mistakes. Manual checks can be too time-consuming for your team and also leave you open to human error.
Automated quality checks run by tools like SurveyCTO can relieve some of the burden on supervisors to be checking and monitoring data collected by enumerators.
What can cause data quality issues?
There are a number of challenges that can arise when collecting data that can impact data quality.
1. Respondents, enumerators, and research assistants are human: This means they can make errors. Respondents can become tired of answering questions, which can lead to issues like skipped questions, careless answers, or incorrect information recall.
Enumerators also have a difficult role and can face challenges such as reluctant respondents, long travel times, and difficulties accessing respondents.
Research assistants can then find themselves in an uncomfortable middle position where they don’t want to call out inefficiencies. They are often also working with huge time and budget constraints.
2. Inconsistent data collection standards: When there are variations in questions and data is not collected using the same protocol across the board, inconsistencies can show up in the research.
This generally occurs when survey questions are not well-planned and the appropriate tests are not performed. Inconsistencies can also arise when team members are not properly trained to follow the procedures for a specific project.
3. Issues in communication between different people and teams: Communication problems can arise for a number of reasons. Some of these include:
- Poorly designed questions or a lack of follow-ups
- Language differences and inadequate translation/interpretation resources
- Geographic limitations like differences in time zones
- Cultural barriers that hinder participation
- Crisis or emergency situations
4. A lack of data assurance processes: Data assurance includes a number of steps you can follow to ensure the quality of your data and monitor it throughout the process. This can keep sound data collection and data entry practices on track. Without a plan, errors are much more likely to occur.
5. Missing tools and resources: Without the correct tools in place, you’re prone to relying too much on manual labor. This leaves you open to error and inefficiencies. It also demands a lot from the wider team, when they could be dedicated to other work.
6. Attrition: When study participants leave that can lead to inconclusive results. This happens in most studies and can create bias in the results if not properly accounted for.
Case studies: See how other organizations achieve data quality
Read through these case studies, which illustrate how other organizations have used SurveyCTO’s quality assurance tools to ensure a high standard of data quality in their research.
- How ATR Consulting used case management to track 14,000 phone surveys about the economic impacts of COVID-19 in Afghanistan
- Pro tips for designing and deploying complex agricultural surveys from IFPRI
- What’s the impact of organized crime on government efforts to fight COVID-19 in Colombia?
Ways you can ensure data quality with SurveyCTO
SurveyCTO is a primary data quality collection tool built with data quality best practices baked into the platform. Here are some of the features you can take advantage of to avoid errors and improve data quality in your field research:
1. Intelligent audits
These audits allow you to monitor the work of everyone on the team in real-time, regardless of their location. SurveyCTO can capture meta-data such as survey timing, response speed violations, audio audits, text audits, background geolocation, device sensor data (light, sound, movement) and more.
2. Smart questionnaire design
There are a few different ways you can put mechanisms in place to reduce respondent errors before they happen. Here are a couple of examples:
- Preloading external data to set default values, which prevents enumerators from re-entering information that has already been collected.
- Create mechanisms that remove the possibility of skip-pattern errors and restrict answers to prevent incorrect answers.
3. Automated quality checks
As we’ve seen, quality checks are an essential part of a solid data quality assurance plan. However, manual quality checks can overburden teams and lead to human error. Planned, automated quality checks allow you to improve the performance of survey questions for better outcomes.
You can use SurveyCTO to automate quality checks by adding your quality criteria and other relevant parameters. The system will then automatically sift through thousands of survey submissions, sending alerts when it detects any potential quality issues.
4. Collaboration tools
With SurveyCTO’s collaboration tools, you can share summaries of form data with external viewers using the Data Explorer. This keeps everyone on the same page. The external viewer feature is also helpful for promoting collaboration. With the review and corrections workflow, you can also combine fieldwork monitoring with quality control actions.
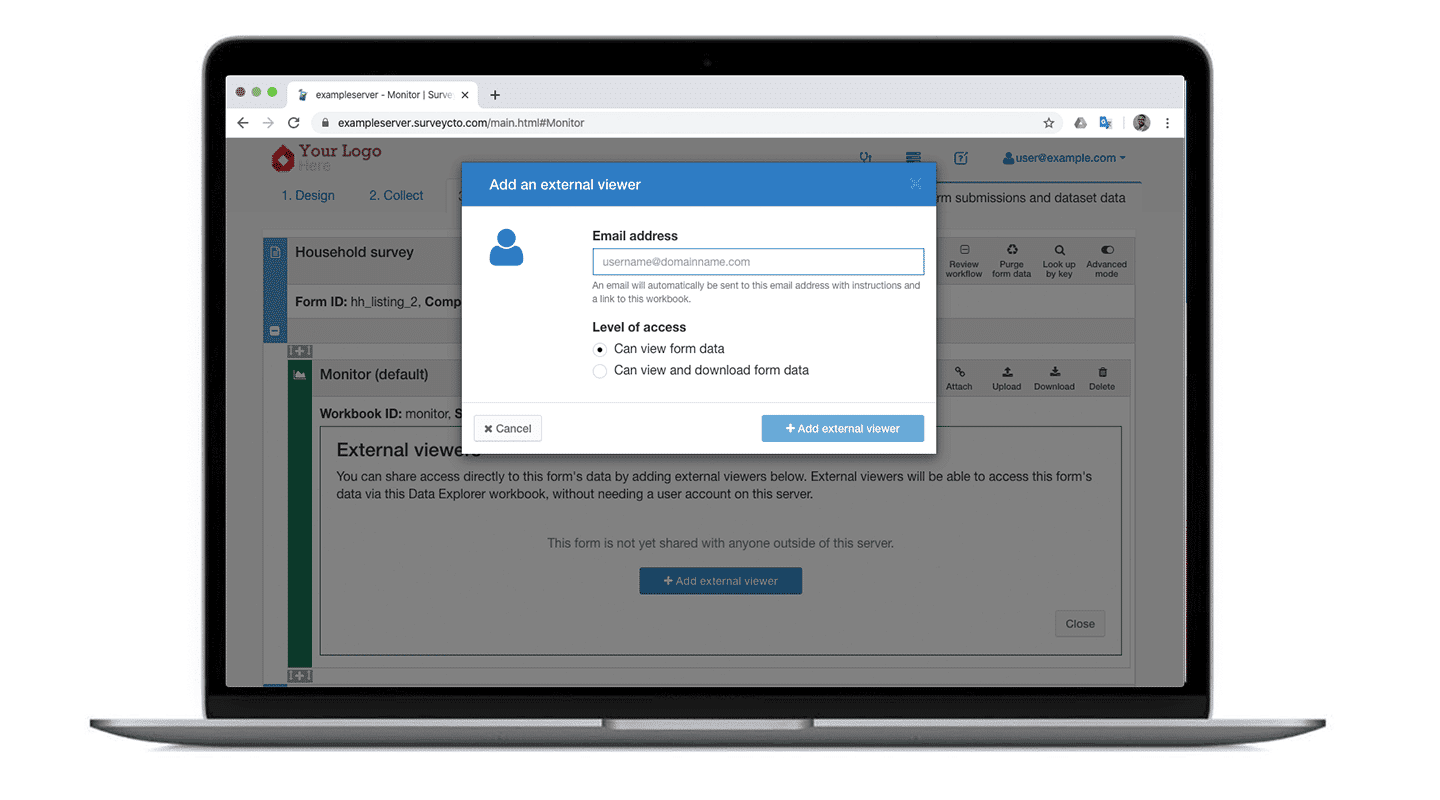
5. Flexible data export and review
SurveyCTO gives reports that let you review data in real-time. You also can send incoming data straight to Google Spreadsheets or any other platform, as well as merge exporting data with Excel spreadsheets. Every time you export data, you’ll also receive a companion report that summarizes key data quality indicators.
6. Easy back check management
To avoid printing, re-uploading, and trying to find surveys for back checks, you can pre-load data to compare the back-checked data with the original.
Check out this post for even more information on how SurveyCTO can help enhance your data and take a look at our quick start guide to help get started with ensuring quality data.
Bottom line: ensuring data quality takes preparation, planning, and monitoring
High quality data is crucial. This is whether you’re using data to inform an important decision, trying to get buy-in or funding, want to make changes, or if you’re trying to understand more about a certain group of people.
Ensuring data quality takes preparation, planning ahead, and monitoring. It’s not always easy, but with the right systems and tools in place, you can ensure that your data quality will help you achieve your research aims.
Your next steps: Explore more resources
To keep learning about methods and best practices for ensuring data quality, take advantage of these resources from SurveyCTO:
- Get emails about our monthly webinars, where researchers from organizations like The World Bank and IDinsight showcase primary data collection methods and best practices. Sign up here to get notified about those webinars.
- Check out previous webinars from SurveyCTO about data quality, like this one on high-frequency checks.
- Sign up for a free trial of SurveyCTO for your next primary data collection project (no credit card required).
To see how SurveyCTO can help ensure data quality, start a free 15-day trial today.